Big Data Querying using Partitions
- Biyi Akinpelu
- Apr 15, 2023
- 6 min read
To query Big Data using SQL, you typically need to use a distributed data processing system that can handle the large volumes of data.
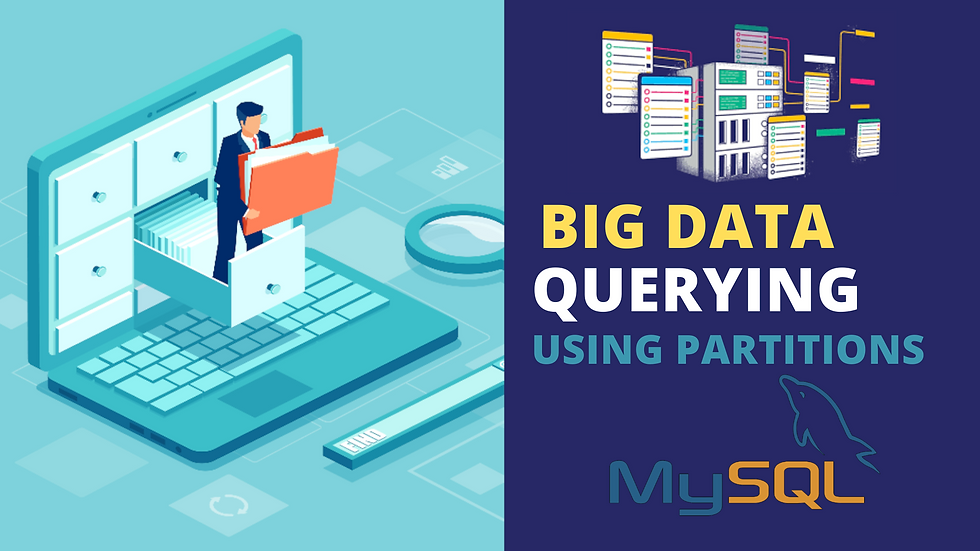
Big Data Querying Alternatives
Here are some common approaches for querying Big Data using SQL:
Distributed SQL databases: These databases are designed to handle large volumes of data and distribute processing across multiple nodes. Examples include Apache Cassandra, Apache HBase, and Google Cloud Spanner.
SQL-on-Hadoop: This approach involves running SQL queries on data stored in a Hadoop cluster, using tools like Apache Hive, Apache Impala, or Presto.
Cloud-based data warehousing: Cloud providers like Amazon Web Services, Microsoft Azure, and Google Cloud Platform offer data warehousing solutions that can handle large volumes of data and allow you to run SQL queries on the data using tools like Amazon Redshift, Azure Synapse Analytics, or Google BigQuery.
Distributed query engines: These tools are designed to run SQL queries across multiple data sources and nodes, allowing you to combine data from different sources. Examples include Apache Drill, Apache Spark SQL, and Presto.
When querying Big Data using SQL, it's important to optimize your queries for performance and to be aware of any limitations or constraints of the system you're using. This may involve using techniques like partitioning, indexing, and caching to speed up queries, and being mindful of the amount of data you're retrieving and the resources required to process it. We will now discuss a little further how to approach querying Big Data with MySQL database.
Querying Big Data with MySQL
In order to query Big Data with MySQL, you will need to create multiple databases running concurrently using with mysql replication functions and optimizing the MySQL databases to handle big data, which can involve several steps to improve performance and scalability.
Here are some general tips for optimizing MySQL to handle up to 1 million records:
Indexes: Create indexes on the columns used in WHERE, JOIN, and ORDER BY clauses to speed up queries. However, avoid creating too many indexes as they can slow down data inserts and updates.
Partitioning: Partitioning can help split large tables into smaller, more manageable pieces. It can also help with performance by allowing queries to only access the relevant partitions.
Query optimization: Optimize your queries to use efficient join algorithms, minimize the number of queries, and use the EXPLAIN command to analyze and optimize your queries.
Hardware resources: Ensure that your server has enough resources to handle the data load. This may involve upgrading the RAM, CPU, and disk storage capacity.
Caching: Use caching techniques like query caching and caching results in memory to speed up queries.
Monitoring and maintenance: Monitor your MySQL server for performance issues and optimize your server settings, such as the buffer pool size and query cache size.
Use a database administrator (DBA): A DBA can help optimize your MySQL server and provide best practices for handling big data.
It's important to note that the specific optimizations required for handling over 1 million records in MySQL will depend on factors such as the complexity of your data, the types of queries you're running, and the available hardware resources.
A Practical Approach to Querying Big Data with MySQL
Here are some practical steps to take to querying Big Data using Partitions with MySQL:
1. Creating Indexes:
-- Creating an index on a column:
CREATE INDEX idx_customer_name ON customers (customer_name);
-- Creating a composite index on multiple columns:
CREATE INDEX idx_customer_order ON orders (customer_id, order_date);
2. Partitioning:
-- Creating a partitioned table:
CREATE TABLE orders (
order_id INT NOT NULL,
order_date DATE NOT NULL,
customer_id INT NOT NULL,
total_amount DECIMAL(10,2) NOT NULL,
...
)
PARTITION BY RANGE (YEAR(order_date))
(
PARTITION p0 VALUES LESS THAN (2010),
PARTITION p1 VALUES LESS THAN (2011),
PARTITION p2 VALUES LESS THAN (2012),
PARTITION p3 VALUES LESS THAN MAXVALUE
);
3. Query optimization:
-- Using efficient join algorithms:
SELECT *FROM customers
JOIN orders USING (customer_id)
WHERE customer_name = 'John Smith';
-- Minimizing the number of queries:
SELECT COUNT(*) FROM orders WHERE order_date >= '2022-01-01';
SELECT SUM(total_amount) FROM orders WHERE order_date >= '2022-01-01';
-- Analyzing and optimizing queries using EXPLAIN:
EXPLAIN SELECT *FROM customers
JOIN orders USING (customer_id)
WHERE customer_name = 'John Smith';
4. Hardware resources: Optimizing hardware resources may involve upgrading your server's RAM, CPU, and disk storage capacity. Here is an example of increasing the buffer pool size in MySQL:
-- Increasing the buffer pool size to 2GB:
innodb_buffer_pool_size=2G
5. Caching: MySQL has a query cache that can be used to cache frequently used SELECT statements. Here's an example of enabling and disabling the query cache:
-- Enabling the query cache:
query_cache_type=1
query_cache_size=128M
-- Disabling the query cache:
query_cache_type=0
6. Monitoring and maintenance: MySQL provides several system variables that can be adjusted to optimize server performance. Here's an example of adjusting the innodb_log_file_size variable:
-- Increasing the size of the InnoDB log files:
innodb_log_file_size=512M
7. Use a database administrator (DBA): A DBA can help optimize your MySQL server and provide best practices for handling big data. Here's an example of a DBA monitoring the slow query log:
-- Analyzing the slow query log:
mysqldumpslow /var/log/mysql/mysql-slow.log
These are just a few examples of the types of code optimizations you might make when working with big data in MySQL. The specific optimizations required will depend on the specific needs of your application and data.
Querying using Partitions
Partitioning is a technique used in MySQL to split a large table into smaller, more manageable pieces called partitions. Each partition is stored separately and can be queried independently, which can improve query performance and make it easier to manage large datasets. Partitioning is commonly used with tables that have billions of rows, as it allows for faster data retrieval and reduces the amount of data that needs to be processed in a single query.
To query data with partitions, you can use the same SQL syntax that you would use to query a regular table. However, instead of querying the entire table, you can specify which partitions you want to query by using the PARTITION keyword. For example, to query only the data in the partition for the year 2022 in a partitioned table called orders, you could use the following SQL statement:
SELECT * FROM orders PARTITION (p2022) WHERE order_date BETWEEN '2022-01-01' AND '2022-12-31';
Querying using Replication
MySQL replication is a technique used to duplicate a MySQL database across multiple servers. This can improve application performance by spreading the load of incoming requests across multiple servers, and it can also provide redundancy in case of a server failure. Replication works by copying the data from a primary server to one or more secondary servers, which can then be used to handle read-only queries.
To use replication with MySQL, you first need to set up a primary server and one or more secondary servers. You then configure the primary server to replicate its data to the secondary servers by setting up replication channels. Once replication is set up, the primary server will automatically send all changes to the secondary servers, which can then be used for read-only queries.
To perform a read-only query on a replicated MySQL database, you can connect to any of the secondary servers and issue a SELECT statement. The secondary servers will have a copy of the data from the primary server, so they can be used to handle read-only queries without affecting the primary server. However, it's important to note that writes (INSERT, UPDATE, DELETE) should only be performed on the primary server, as changes made on the secondary servers will not be replicated back to the primary server.
A Replication Approach
Here's a short practical example for setting up replication in MySQL:
Assuming we have a primary server (hosted at primary.example.com) and a secondary server (hosted at secondary.example.com), we can set up replication as follows:
1. On the primary server, create a new user account that will be used for replication:
CREATE USER 'replication_user'@'%' IDENTIFIED BY 'password'; GRANT REPLICATION SLAVE ON *.* TO 'replication_user'@'%';
2. On the secondary server, create a new user account that will be used to connect to the primary server:
CREATE USER 'repl_user'@'primary.example.com' IDENTIFIED BY 'password';
3. On the primary server, take a backup of the database that we want to replicate (let's call it mydatabase). This can be done using the mysqldump tool:
mysqldump -u root -p mydatabase > mydatabase.sql
4. Copy the backup file to the secondary server:
scp mydatabase.sql repl_user@secondary.example.com:/tmp/
5. On the secondary server, restore the backup using the mysql command:
mysql -u root -p mydatabase < /tmp/mydatabase.sql
6. On the secondary server, set up the replication by running the following SQL commands:
CHANGE MASTER TO MASTER_HOST='primary.example.com', MASTER_USER='replication_user', MASTER_PASSWORD='password'; START SLAVE;
7. The secondary server should now be replicating data from the primary server. To verify that replication is working, you can run the following command on the secondary server:
SHOW SLAVE STATUS\G
This will show the current replication status, including the position in the replication stream and any errors that may have occurred.
Recommended Book on Big Data and MySQL
MySQL 8 for Big Data
Effective data processing with MySQL 8, Hadoop, NoSQL APIs, and other Big Data tools is a book that describes how to query very large data usually referred to as Big Data. It was written by Shabbir Challawala, Jaydip Lakhatariya, Chintan Mehta, Kandarp Patel. The book presents several practical approaches that can be used to solve problems querying Big Data elegantly, and effectively. It is considered as a classic reference in the field of Database Programming and Engineering.
Comments